We are going through one of the most divisive presidential campaigns in the modern era. Both candidates and their campaigns claim catastrophe if the other were to get elected. And they are focusing on distinct topics to make that claim. For instance, while Joe Biden states that democracy and decency are on the ballot, Trump suggests it is law and order. The question is: are their narratives sticking? In a new collaboration with CNN and SSRS, we are starting to answer these questions. A short overview of our methodology that relies on weekly surveys can be found here. And some of our early reflections have been shared here.
In this blog post, I am going to build on these findings to hopefully add more details that might interest politics aficionados and to highlight text analysis techniques that can be leveraged to look at our data from a slightly different perspective. We will have one main goal in mind in this blogpost: highlighting contrasts. After all, as I mentioned when starting this blog, this is what the campaigns are aiming to do.
How to identify contrasts?
Be it the distinctions between what people say about Biden vs. Trump or between what different demographic groups say about each candidate, drawing distinctions boils down to comparing two corpora.
Take, for instance, the distinctions between Trump and Biden. Various natural language processing (NLP) approaches can be used to compare these two corpora to identify their differences. We can start with the simplest approach. One can simply look at the most common words (and indeed some of the analysis already shared on CNN does exactly this). Of course, this does not necessarily provide unique words, just the most popular words. Such an analysis can mask important distinctions by being dominated by generally popular words. Luckily for us, these common words were already rather different for the two candidates, naturally revealing some important differences (see the CNN article linked above). But generally speaking, one needs to build on these simple frequencies to start to understand the differences, for instance by computing the differences in frequencies, the ratio of frequencies or the log odds ratio. Unfortunately, these approaches do not work too well for rare words. Here, we turn to log-odds with informative Dirichlet priors, as introduced by Monroe et al. (2016) as a helpful alternative. This approach uses a third (background) corpus to shrink the estimates based on a prior given by this background corpus, providing better estimates for rare words. The difference in usage of a word w in corpus i (e.g. responses to “read, seen or heard” about Trump) and j (e.g. responses to “read, seen or heard” about Biden) is as follows using this technique:
where fwi is the frequency of word w in corpus i, fwj is the frequency of word w in corpus j, αw is the frequency of word w in the background corpus, ni is the size of corpus i, nj is the size of corpus j, and α0 is the size of the background corpus. We next account for our certainty about those estimates using variance and computing the z-scores for each word w.
What is uniquely defining Trump and Biden?
We start examining contrasts with the most basic one: Trump v. Biden. In other words, we ask: “which words more uniquely describe Trump (and Biden)?”. We use the survey responses from July 12 to September 1, 2020. Using this dataset, we construct two corpora: Responses to “read, seen or heard” about Trump and responses to “read, seen or heard” about Biden. Ideally. The background corpus should be a third, and large, corpus that can be used to determine our priors but here we will simply use corpora 1+2 (we will change our background corpus in later iterations of figures, stay tuned!) Having defined our corpora, we are now ready to employ the approach introduced by Monroe et al. and find words that uniquely define the two candidates according to our respondents. Here it goes…
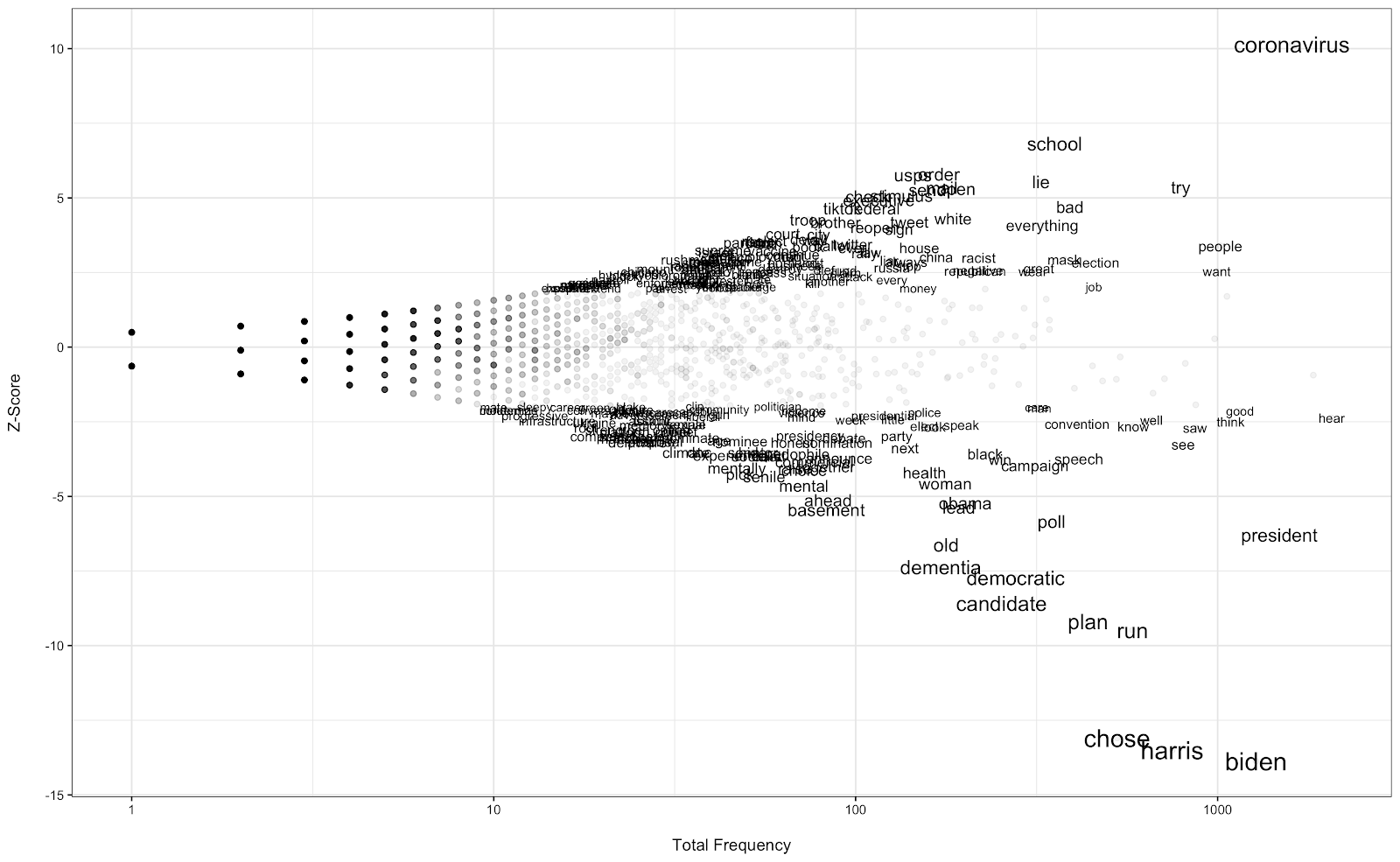
I am using the x-axis to denote overall popularity of the word in our surveys. On the y-axis, I am providing the z-score. Note that words with z-scores in the range of [-1.96, 1.96] are only represented by a dot in this plot, for ease of viewing. Such words are not significantly unique to one corpus. A couple of insights get revealed immediately. First, we observe that coronavirus is top-of-mind for respondents when talking about Trump. VP pick is top-of-mind for Biden. We also see a distinction between the negative topics that stuck for the two candidates. While words like “old” and “dementia” are more uniquely associated with Biden, words like “lie” and “racist” are associated with Trump. One other point to keep in mind: respondents are referring to Biden by name when discussing the candidate while this is not as common for Trump. Indeed, the z-score for “trump” was negative (though not significant). Meaning that that word was more commonly seen in responses given about Biden. This could be because the respondents are more concerned about Trump and are focusing on these concerns when discussing Biden.
How does the party affect what people say about the candidates?
We can use the technique described to next investigate if Democrats and Republicans are remembering similar different words when discussing the candidates. This is exactly what we do.
Let’s start with Biden. The two corpora compared are Republican and Democrat responses about Biden. The background corpus includes all responses (i.e. including Independents etc.). The analysis results are below.
Positive z-scores denote words that are more commonly used by Republican respondents (and negative ones are used by the Democrats). Important distinctions are revealed. Some of these are obvious–Republicans use pejorative words and focus on Biden’s age while Democrats are focusing on positive words. Also note the word ‘pedophile’, which might be related to conspiracy theories spreading online. There are also less obvious patterns that emerge. Democrats have a stronger focus on policy words (e.g. economy) and on the pandemic. We also see further support of the anti-Trump focus respondents have when talking about Biden–Trump is more commonly evoked by the Democrats when talking about Biden.
Now, let’s turn to responses about Trump. The plot below shows the result of a similar analysis described for Biden above.
Here, we see a similar pattern. Republicans are using significantly more positive words compared to Democrats. Republicans focus on the media (medium after lemmatization) and the Democrats, more so than the Democrat respondents. They also focus on the economy more when discussing Trump. Interestingly, coronavirus mentions are not significantly different across the two parties. This raises an important point: Assuming that the Republicans are generally supportive of the candidate of their party, this suggests that not all mentions of covid-19 were negative in sentiment. Finally, Democrats also have a unique focus on Trump’s golfing habits and the news about the USPS, highlighting a few scandals that might be sticking (at least so far).
There are various other ways to slice our data and examine contrasts that emerge. But I will limit our analysis to the three comparisons listed above in this blog. There are also various other techniques to apply to examine this rich dataset. And apply we will! For now, I hope you enjoyed this short blog post about our Election 2020 collaboration. Stay tuned for future analysis and updates from us. Check out our website for changes to the plots provided above as we collect more data in the upcoming weeks.
References:
Monroe, B.L., Colaresi, M.P. and Quinn, K.M., 2008. Fightin’words: Lexical feature selection and evaluation for identifying the content of political conflict. Political Analysis, 16(4), pp.372-403.